Advancing Geotechnical Engineering with AI - Bayesian Back Analysis for Settlement Prediction of Soft Soils
- levonrush
- May 14, 2024
- 2 min read
As an AI developer and product manager, I am thrilled to share my work on an innovative project with Merrick Jones, a PhD candidate at the University of Newcastle, Australia. Our project, "Bayesian Back Analysis for Settlement Prediction of Soft Soils," aims to revolutionise the way geotechnical engineers predict settlement in soft soil areas, particularly for infrastructure projects such as road construction.
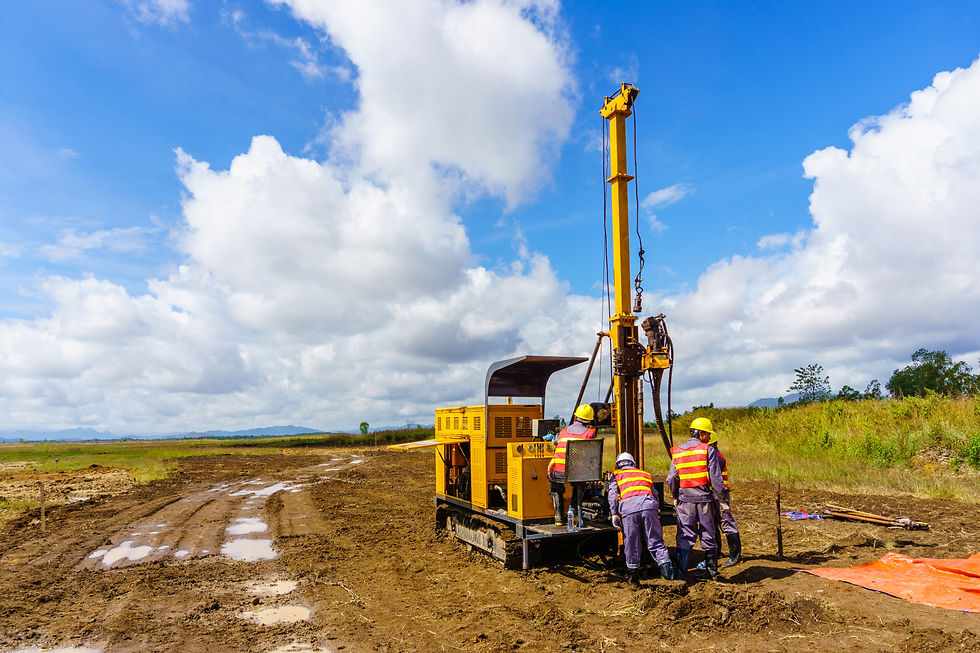
Project Overview
Population growth along the coast of eastern Australia has driven the demand for new and upgraded transport infrastructure. This has introduced challenges in road foundation design, particularly in coastal floodplains and hinterland areas underlain by soft soils. These soils, characterized by low stiffness, shear strength, and permeability, pose significant risks such as embankment instability, excessive ground settlement, and prolonged construction times.
The Bayesian Back Analysis Method
Traditional methods for predicting soil settlement, such as the Asaoka and hyperbolic methods, have limitations, especially when dealing with the variability of soft soil profiles. Our project addresses these challenges by developing a Bayesian Back Analysis Method, which offers a more accurate and efficient approach to predicting settlement.
Key Innovations:
Advanced Constitutive Modeling: Incorporating features like anisotropy, destructuration, and time-dependency to better model the behavior of soft soils.
Effective Numerical Algorithms: Combining coupled consolidation analysis with Bayesian techniques to improve prediction accuracy.
Modeling Spatial Variability: Using random field theory to account for the inherent variability in soil properties.
Efficient Inverse Algorithms: Implementing a modified BUS approach to enhance computational efficiency.
Utilizing Cloud Computing: Leveraging cloud resources to perform complex simulations more efficiently.
Application to Real-World Projects: Applying our methods to real projects, such as those at the National Field Testing Facility at Ballina, NSW.
My Role
In this project, I am working closely with Merrick Jones to develop a modern, automated, and deployable machine learning platform. This platform will enable Merrick and other researchers to efficiently work on the project backend, continuing the development and refinement of the settlement prediction algorithm.
Project Impact
Our Bayesian Back Analysis Method offers several advantages:
Early Decision Making: Providing reliable predictions early in the construction process, allowing for timely risk management and cost savings.
Enhanced Accuracy: Offering more precise settlement predictions by considering multiple soil layers and complex fill staging.
Improved Industry Practices: Helping government agencies like Transport for New South Wales rigorously evaluate road designs in-house, improving the quality of infrastructure projects.
Conclusion
By integrating advanced AI techniques with geotechnical engineering practices, our project aims to significantly enhance the accuracy and reliability of settlement predictions for soft soils. This not only benefits the construction industry by reducing costs and improving project timelines but also contributes to the overall safety and sustainability of infrastructure development.
Stay tuned for more updates on this exciting project, and feel free to reach out to learn more about how AI can transform geotechnical engineering practices.
Comments